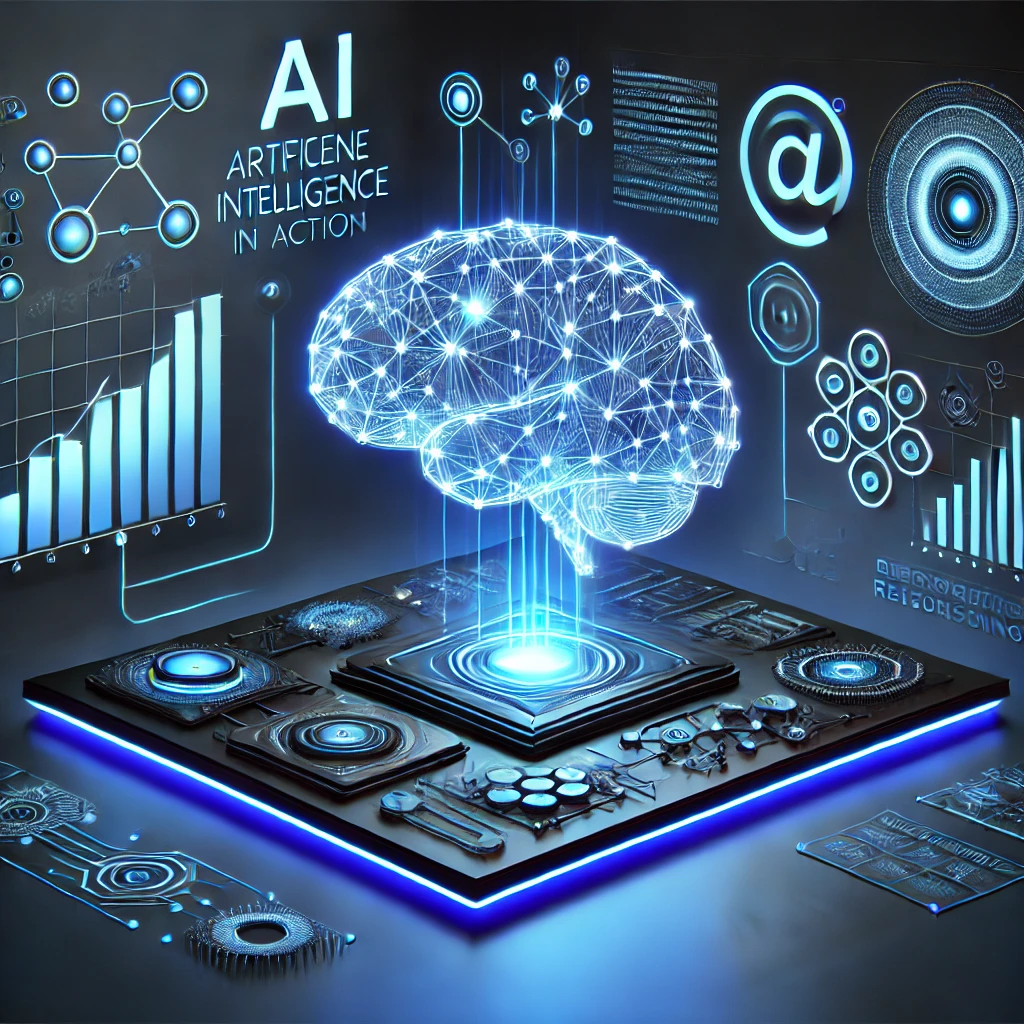
The team at OpenAI has been celebrating the holiday season with a playful take on the 12 Days of Christmas. So far, we've seen updates showcasing how GPT Pro is solving multimodal challenges and announcements like the rollout of SORA, but one update that stood out to me was the expansion of their Reinforcement Fine-Tuning (RFT) program.
What is Reinforcement Fine-Tuning (RFT)
RFT is a training-data-efficient method that fine-tunes AI models for specific domains. Unlike traditional fine-tuning methods that require vast amounts of labeled data, RFT works with as little as a few dozen training examples. This efficiency stems from its unique approach:
The model is encouraged to "reason" through problems, generating answers based on logical steps.
Correct reasoning paths are reinforced, while flawed approaches are penalised. This iterative process doesn't just teach the model what to think but how to think better within a specific context. The result? A smaller, fine-tuned model (like OpenAI's o1-mini) can outperform larger, more resource-intensive models (such as o1) in specialised tasks. RFT is the approach OpenAI uses to tune their frontier models.
A Case in Point: Genetic Research
During the update on day two, OpenAI shared a compelling example of how RFT is being applied to genetic research. Using RFT, a fine-tuned o1-mini model surpassed the larger o1 model in analysing case reports and identifying genes linked to specific symptoms. This highlights the transformative potential of RFT—not just for performance but for cost-efficiency and accessibility in research-heavy domains.
One of the most significant parts of the session was how OpenAI is making RFT more accessible by removing most of the operational complexity. OpenAI's RFT automation tools simplify the process, enabling researchers, universities and enterprise teams to focus on what matters most: curating data and selecting appropriate grading mechanisms.
For many organisations, especially those without robust machine-learning capabilities, this latest platform feature lowers the barrier to entry and unlocks new opportunities to harness domain-specific AI.
The Future of Domain-Specific AI
Maximising value from large language models isn't just about choosing the most advanced frontier models—it's about making them perform exceptionally with domain-specific data, efficiently and at scale. While RFT is just one approach, I expect other providers and open-source projects to focus on automating and simplifying the fine-tuning process in 2025.